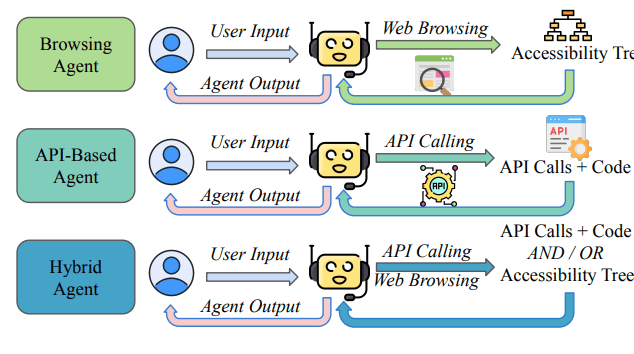
Top 5 AI Agent Papers of 2025
1. Beyond Browsing: API-Based Web Agents
Paper: “Beyond Browsing: API-Based Web Agents” (2025)
Key Idea
Traditional web agents rely heavily on browser-based automation. This paper proposes a hybrid approach—an agent that can seamlessly switch between direct API calls and browser interactions to achieve web tasks. The authors show significantly higher success rates on the WebArena benchmark (up to ~24% improvement) when using the hybrid strategy vs. browser-only agents.
Why It Matters
If you’re building or scaling web automation systems (shopping bots, data-mining agents, or more advanced “personal assistants”), these insights will help you enhance performance. Using APIs wherever possible—and gracefully falling back to a browser when necessary—both speeds up interactions and reduces error rates.
2. Cocoa: Co-Planning and Co-Execution with AI Agents
Paper: “Cocoa: Co-Planning and Co-Execution with AI Agents” (2025)
Key Idea
Cocoa reframes human–AI collaboration: users and agents build an action plan together and then share execution steps. This fosters better human oversight and trust.
Why It Matters
When building multi-step or research-heavy workflows (think content generation, code refactoring, design tasks), having a structured environment—rather than mere conversation—lets your users maintain fine-grained control. Cocoa’s approach can be integrated into your own “doc-based” or “IDE-based” collaboration tools.
3. AutoAgent: A Fully-Automated and Zero-Code Framework for LLM Agents
Key Idea
AutoAgent is an “Agent OS” that lets non-programmers build and customize LLM agents simply by describing desired goals and available tools in natural language. It automatically orchestrates tool usage, self-management, and self-play—no code required.
Why It Matters
Ever had non-technical colleagues who want an intelligent agent but can’t code? AutoAgent could be your friendly neighbor: provide them a natural-language interface to define tasks, and let the system handle the rest. Especially useful in enterprise settings where domain experts can define their own automated workflows.
4. Magma: A Foundation Model for Multimodal AI Agents
Paper: “Magma: A Foundation Model for Multimodal AI Agents” (2025)
Key Idea
Magma unifies visual, textual, and action-based data to support a single agent that can interpret images, process language, and execute actions (in UIs and even robotics). It achieves state-of-the-art results across diverse tasks—UI automation, robotic manipulation, and standard vision-language benchmarks.
Why It Matters
If your application demands complex user-interface interactions or hardware integration (drones, robots, AR, you name it), a single foundation model that covers multiple modalities can simplify your stack. Magma’s approach promises to unify code, data, and performance improvements under one robust system.
5. WebGames: Challenging General-Purpose Web-Browsing AI Agents
Benchmark Suite: “WebGames” (2025)
Key Idea
WebGames compiles over 50 interactive challenges that mimic real browser tasks. The findings reveal that even leading AI models only solved around 41% of them vs. 95% success by humans.
Why It Matters
If you need a standardized environment to test or stress-test your web automation agent, WebGames is a prime candidate. It offers reproducible tasks and highlights what agents struggle with most (adaptation, dynamic page structure, unpredictable forms). Perfect for identifying your bottlenecks and tracking improvements.
Sample Code Snippet for Hybrid Web + API Agents
Below is a minimal Python snippet showing how you might set up a simple hybrid agent (concept inspired by Beyond Browsing: API-Based Web Agents). It uses a hypothetical library that can switch between a browser-driven approach and direct API calls:
# initialize libraries
from hybrid_web_agent import HybridWebAgent, APIHandler
# setup agent and tools
api_tools = APIHandler(token="your_api_token")
agent = HybridWebAgent(api_handler=api_tools, headless=True)
# define task
task = "Find the best flight deal to NYC and fill in a booking form"
# run agent
agent_result = agent.execute(task)
print("Agent Result:", agent_result)
Final Thoughts
These five works reflect AI’s steady march toward more robust, flexible, and user-friendly agent systems. Whether you’re experimenting with partial automation or building complex enterprise solutions, the latest research offers a wealth of strategies, from zero-code agent frameworks to co-planning paradigms. Keep an eye on them—and consider how to integrate these approaches into your own pipeline.