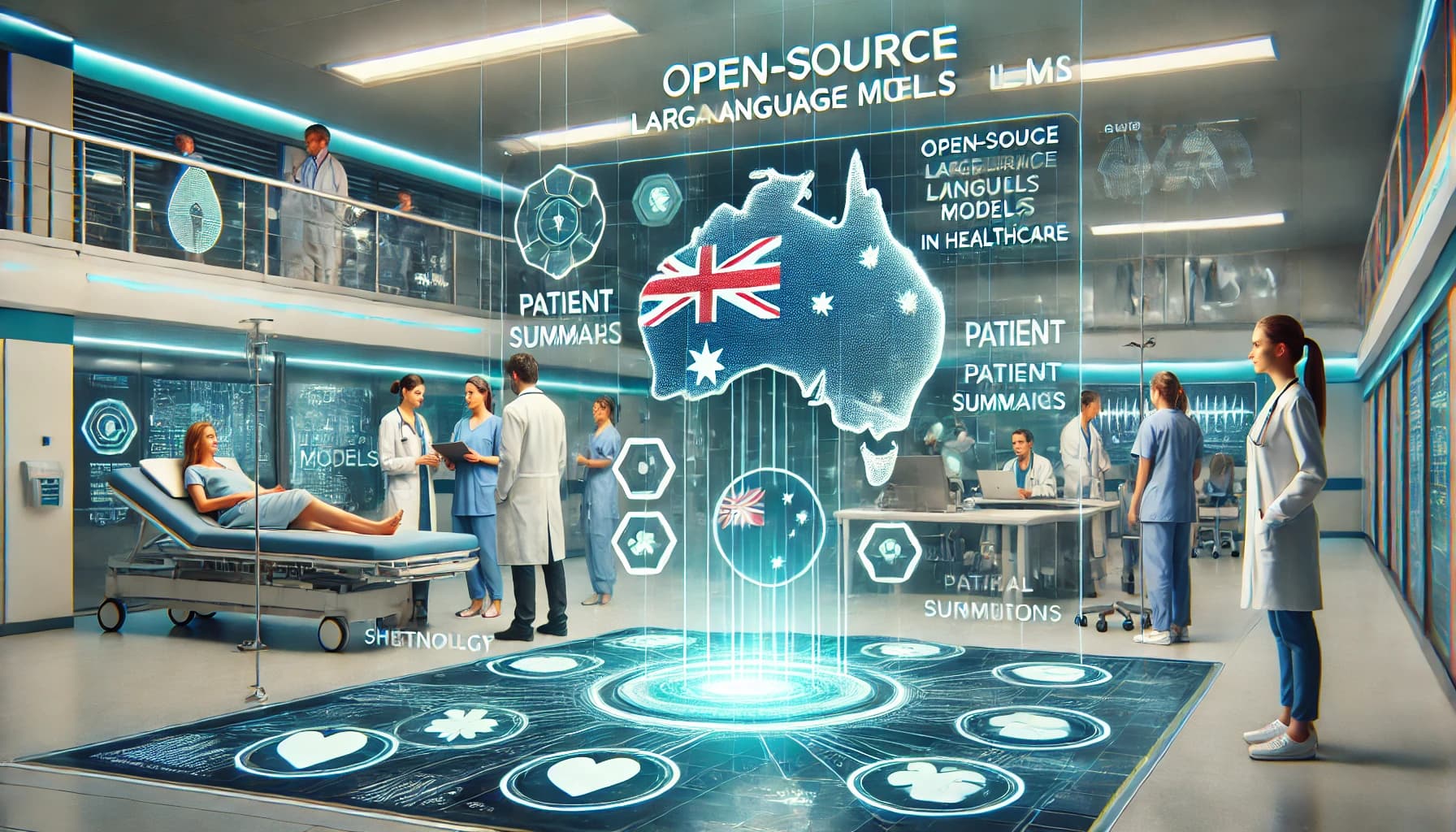
Open-Source LLMs Transforming Australian Healthcare: 4 Real-World Use Cases
Introduction: Why Open-Source LLMs for Healthcare?
Generative AI is making waves in healthcare. With data privacy concerns and strict regulations, many Australian hospitals and health-tech companies are opting for open-source large language models (LLMs) like Meta's LLaMA 2 or MosaicML's MPT. By running these powerful models on their own infrastructure, they can maintain data sovereignty and security, a critical factor in patient confidentiality. Below are four real-world examples that illustrate how these open-source LLMs drive impactful innovation in Australian healthcare.
1. Multilingual Cancer Information Chatbot
- Overview: A collaboration by Flinders University and Flinders Medical Centre, this chatbot project aims to provide cancer patients with reliable information in multiple languages.
- The Model & Tools: Researchers tested open-source LLMs such as LLaMA 2 and integrated them with a retrieval-augmented pipeline for medical content.
- Strategic Goal: Enhance patient experience by providing accurate, accessible answers about diagnosis, treatment, and symptoms, particularly for non-English-speaking patients.
- Impact: The pilot demonstrated near-zero serious medical errors in English responses. Although some content needed refinement for lower reading levels, the multilingual capacity offers the potential to empower diverse patient communities.
- Read the full paper
2. AI Scribes for Clinical Documentation
- Overview: Telehealth platform Coviu piloted an AI-powered medical scribe to reduce clinicians' administrative burdens.
- The Model & Tools: Coviu fine-tuned an open-source LLM (based on LLaMA 2) to transcribe and summarize doctor-patient conversations, producing formatted notes in near-real-time.
- Strategic Goal: Let doctors focus more on patient care by automating note-taking and record-keeping.
- Impact: Clinicians saved an estimated 10–15 minutes per consultation, reducing time spent on administrative tasks and improving overall workflow efficiency.
- Read more at healthcareitnews.com
3. Triage Assistant for Epilepsy Surgery Candidates
- Overview: The Royal Adelaide Hospital teamed up with the University of Adelaide to identify epilepsy surgery candidates more accurately.
- The Model & Tools: A hybrid pipeline used classic ML to flag high-risk cases, then an open-source LLM (again, a LLaMA-like model) extracted key clinical details from patient notes.
- Strategic Goal: Ensure no potential candidates for epilepsy surgery slip through the cracks, improving patient outcomes.
- Impact: The AI-based approach delivered accurate, concise summaries, identifying more than half of flagged patients as good surgery candidates, potentially streamlining critical medical interventions.
- Read the full paper
4. Summarizing Aged Care Records to Spot Risks
- Overview: The University of Wollongong and Australian e-Health Research Centre (CSIRO) deployed an open-source LLM in multiple aged care facilities across New South Wales to identify malnutrition risks.
- The Model & Tools: They fine-tuned LLaMA 2 with retrieval-augmented generation to parse thousands of unstructured nursing notes.
- Strategic Goal: Provide more proactive care by automatically flagging seniors at nutritional risk.
- Impact: The AI system's summaries aligned closely with human experts' findings. A prior manual audit that took weeks was completed in mere days, demonstrating massive efficiency gains and the potential for early, life-improving interventions.
- Read the full paper
The Strategic Edge: Why Open-Source LLMs Matter
- Data Sovereignty: By hosting models locally, organizations keep sensitive patient information under their control.
- Customization: Fine-tuning open-source LLMs on domain-specific Australian medical data ensures context relevance and higher accuracy.
- Regulatory Compliance: On-prem solutions make it easier to comply with Australian regulations like the Privacy Act 1988.
- Cost and Scalability: Avoiding heavy usage fees from external APIs can offer more predictable cost structures for healthcare providers.
Executive Takeaways and Next Steps
- Invest in On-Prem Infrastructure: Whether it's private cloud or a secure hybrid model, robust data pipelines are essential for deploying AI at scale.
- Build Clear Governance: Align with governance frameworks that address data privacy, model explainability, and accountability.
- Pilot, Validate, Scale: As shown in these cases, starting with pilots allows teams to prove value quickly before rolling out more broadly.
- Stay Future-Ready: Open-source LLMs evolve rapidly, offering better performance and smaller footprints. Keeping an agile mindset helps organizations integrate the latest innovations.
Conclusion
Open-source LLMs are leading a silent revolution in Australian healthcare. From multilingual patient support to automated documentation and risk prediction, these solutions can unleash major efficiency gains and deliver tangible improvements in patient outcomes. For executives and CTOs, embracing open-source AI is not just a technological decision but a strategic one-one that helps maintain competitive advantages, strengthen data governance, and continually improve the healthcare journey for all Australians.