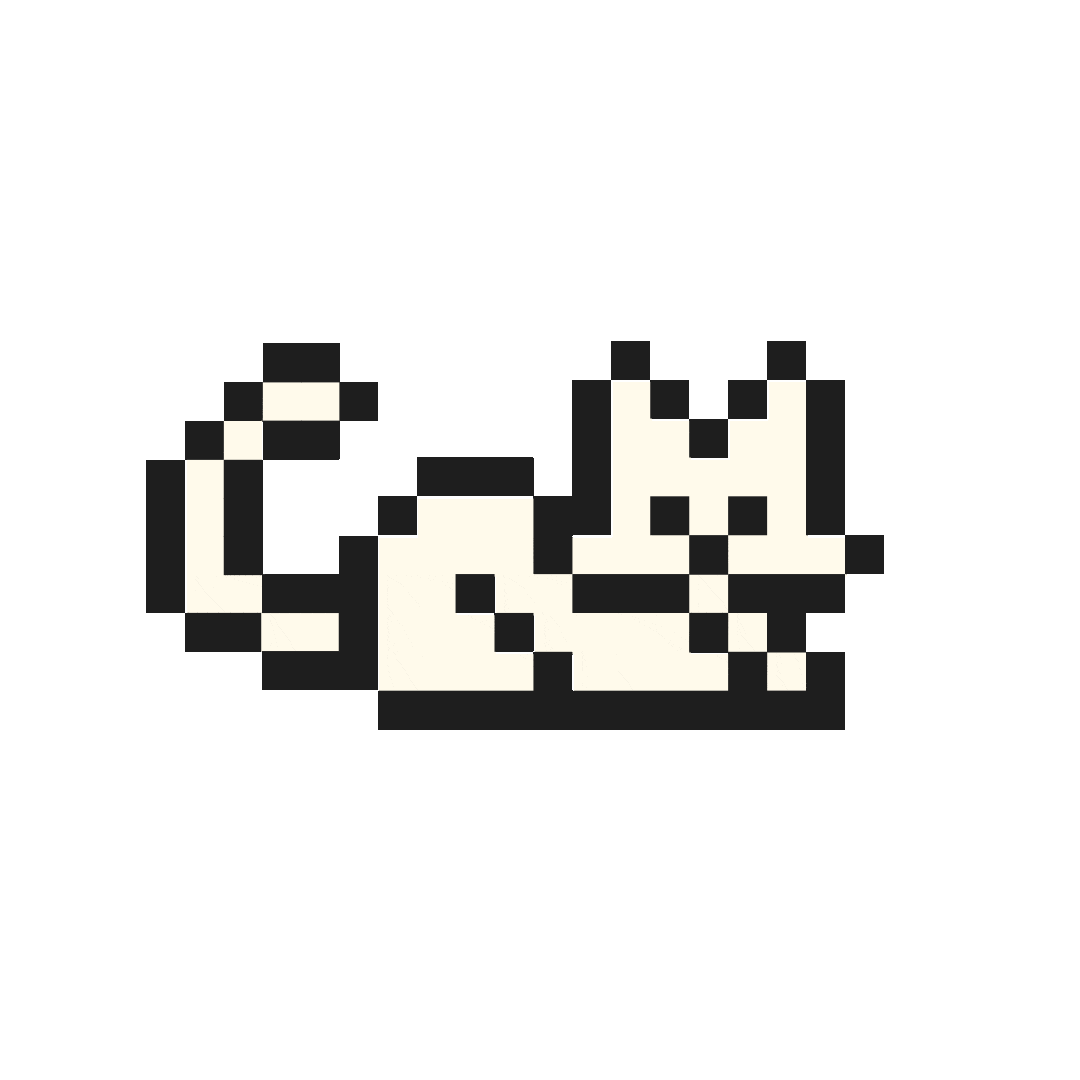
Mistral’s MoE Models in the Real World: Case Studies and Key Insights
Open-source AI is witnessing a dramatic rise in sparse Mixture-of-Experts (MoE) architectures, and Mistral has emerged as a leading name in this space. From enterprises reducing fraud losses to researchers probing memory bandwidth, Mistral’s MoE models are pushing the boundaries of efficiency and capability. Below is a look at how these models are being adopted across industries, academia, and the open-source community—along with lessons learned along the way.
1. Enterprise Success Stories
Finance (Fraud Detection)
A European investment bank integrated Mixtral 8x7B to analyze real-time transaction patterns. Within months, fraud detection accuracy hit ~95%, slashing operational costs by ~30% compared to proprietary APIs. Self-hosting also addressed data-privacy requirements for sensitive financial data.
Healthcare (Drug Discovery)
In drug research, Mistral 7B helped a pharmaceutical company parse massive sets of research papers. This reduced data-analysis times by nearly 40%. Because the model is open-source, the team could inject specialized medical knowledge—yielding more relevant discoveries.
E-Commerce (Customer Support)
A French luxury retailer fine-tuned a Mistral-based chatbot called “Le Chat” and automated 70% of inbound support. Response times fell from about 2 minutes (human agent) to 10 seconds, thanks to Mistral’s multilingual capabilities. This greatly cut support costs and improved customer satisfaction.
Journalism (Content Generation)
Agence France-Presse (AFP) uses Mistral’s MoE architecture for real-time fact checking in AI-produced news content. By cross-referencing internal databases, AFP reduced misinformation risk and sped up routine news summary production. The open architecture also allowed them to integrate verified data directly into the model’s knowledge base.
Databricks reports that as of early 2024, nearly 1,000 enterprises have tested Mistral’s models, logging millions of inferences—underscoring broad adoption across multiple verticals.
Reference: Databricks invests in Mistral AI and integrates Mistral AI’s models
2. Academic Research and Benchmarking
MoE-CAP Study
Researchers in MoE-CAP (2024) discovered that Mixtral-8x7B is often memory bandwidth-bound on standard GPUs (e.g., A100/A6000). On newer, higher-bandwidth cards (H100), inference speeds increase significantly. These findings help organizations optimize hardware choices when scaling MoE deployments.
Multilingual & QA Research
Academic labs have used Mistral’s base models as benchmarks for multilingual tasks and question answering, where Mixtral 8x7B scored 18/18 on professional German certification questions. Researchers point to Mistral’s strong cross-lingual skills as a standout advantage, rivaling (and sometimes surpassing) dense models that are two to three times larger.
MoE Internals
Interest is growing in understanding how gating networks allocate tasks to different experts. Balancing token load and preventing expert “overload” remains a core challenge. Early results suggest Mistral’s top-2 gating recipe handles load reasonably well, but fine-tuning such models still requires cautious monitoring of router behavior.
3. Open-Source Community Projects
Ecosystem Integration
Upon Mixtral 8x7B’s release, Hugging Face quickly supported the model with official weights and library updates for MoE layers. Developers were able to run inference using standard frameworks or specialized backends like Text Generation Inference—spurting a wave of hobbyist fine-tunes, instruction-tuned variants, and multilingual spin-offs.
Community Fine-Tunes and Merges
Within days, the community released Mixtral-8x7B-Instruct-v0.1, a high-quality instruction-tuned model. Merges with other architectures (e.g., Synthia) led to hybrid LLMs that local testers found could surpass many 30B–70B dense models in multilingual tasks. Enthusiasts on Reddit deemed the results “hype well-deserved.”
Tools and Deployment
Open-source groups like Meetrix.io introduced one-click AWS AMIs for Mixtral, enabling fast setup for businesses wanting minimal MLOps overhead. Community developers also explored 4-bit quantization, letting smaller GPU rigs run a 47B-parameter model—though requiring trade-offs in output quality.
Limitations and Fixes
Early adopters noted suboptimal throughput on certain inference engines (e.g., ~15 tokens/s on a 2×A100 server) until MoE-specific optimizations were implemented. Community patches soon boosted speeds by ~50%. Users also reported minor issues with the 32k context window, prompting library updates to address edge cases at high context lengths.
4. Technical Strengths in Practice
1) Efficiency and Inference Speed
By activating only two of its eight experts per token, Mixtral 8x7B runs at the cost of roughly a 13B dense model—yet its total parameters reach ~47B. Benchmarks show that it can match or outperform Llama 2 70B or GPT-3.5 while generating text much faster.
2) Strong Performance vs. Larger Models
Mixtral consistently surpasses many dense models in tasks like multilingual QA and code generation. Some fine-tuned variants score on par with GPT-3.5. For enterprises, this cost-to-performance ratio is often cited as a key reason to choose Mistral over proprietary APIs.
3) Long Context Window
Mixtral’s 32k token context window is a boon for large-document analysis, multi-turn chat, and retrieval-augmented generation. Early testers exploit this to process entire contracts or large knowledge-base articles in a single request, with fewer chunking workarounds.
4) Multilingual and Domain Flexibility
MoE’s inherent “expert specialization” yields wide-ranging strengths. Mistral’s base training spans multiple languages, and it has shown excellent results in coding tasks. One sub-expert might learn to handle code patterns, another is adept at non-English text, and so forth—leading to an adaptable all-in-one model.
5. Ongoing Challenges
Infrastructure & Bandwidth
MoE systems are often memory bandwidth-bound rather than purely VRAM-limited. On GPUs like the A100, Mixtral can saturate memory transfer speeds. Newer hardware (H100) or multi-node clusters may be required to maintain high throughput at scale.
Gating Complexity
Load balancing and router capacity issues add complexity. Fine-tuning data that diverges from training distributions can sometimes overload specific experts, causing token drops. Though Mistral’s top-2 gating helps mitigate this, understanding where mistakes originate (expert A vs. expert B) can complicate debugging.
Memory Footprint
While inference is cheaper than an equivalent dense model, the full 46.7B parameters (or ~90 GB in FP16) must still reside in memory. Many practitioners rely on quantization or multi-GPU sharding to deploy Mixtral. This remains a barrier for smaller teams without high-memory hardware.
Ecosystem Maturity
MoE support in inference frameworks, UIs, and fine-tuning pipelines is rapidly evolving but still catching up. Early adopters sometimes encountered misconfigurations or partial feature support for MoE layers, though community-driven improvements continue to close these gaps.
6. Adoption Trends and Future Outlook
Mistral’s success with sparse expert architectures has amplified interest in MoE LLMs across both open-source and industry. Other players (Google, Alibaba, and independent research groups) are releasing their own MoE variants, building on the traction Mistral demonstrated with Mixtral. The Databricks–Mistral partnership also highlights how collaboration with platform providers can accelerate enterprise adoption and push these models into production-grade deployments.
Performance comparisons frequently show Mixtral 8x7B beating dense models in the 30B–70B range while offering GPT-3.5-level quality. It still lags top-tier models like GPT-4 or PaLM-2 on particularly intricate tasks, but the gap narrows daily. Many organizations opting for on-premise solutions cite Mistral’s open license and flexibility—no vendor lock-in, easy customization, and full data control—as decisive advantages.
As more practitioners confirm the efficiency gains of MoE, we can expect continued innovations in expert routing, memory offloading, and load balancing. Mistral AI is rumored to be testing larger multi-expert designs, aiming to drive further breakthroughs in cost-performance. The consensus among early adopters is that Mistral’s Mixtral 8x7B has validated MoE LLMs in real-world usage—and that these architecturally innovative, high-efficiency models are here to stay.
Final Thoughts
From enterprise fraud detection to multilingual chatbots, Mistral’s MoE models are redefining what is possible with sparse LLMs. While challenges around hardware bandwidth and gating complexity exist, the real-world gains in speed, cost savings, and multilingual proficiency are hard to ignore. By combining open-source accessibility with top-tier performance, Mixtral 8x7B has galvanized a vibrant ecosystem of researchers, developers, and businesses eager to harness MoE technology.
As the AI community continues to refine MoE training techniques and optimization stacks, expect even more impressive results—and far-reaching adoption across an expanding range of domains. The next generation of AI may well be driven by specialized experts working in unison, embodying Mistral’s ethos of power and efficiency.