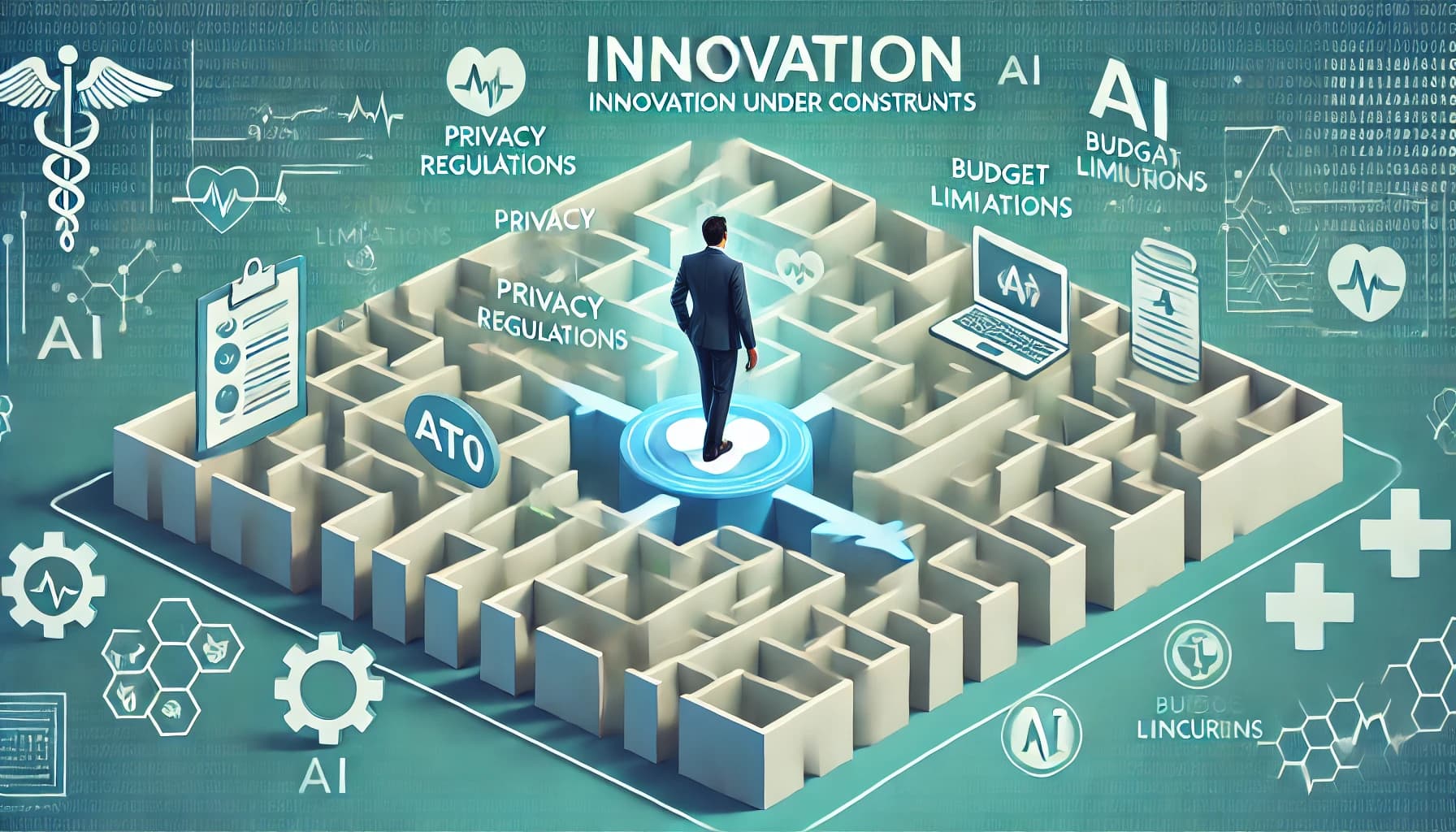
Leading AI Innovation Under Constraints: Strategies for Healthcare CTOs
Australian healthcare CTOs find themselves facing a unique puzzle. On the one hand, AI and machine learning promise to revolutionize patient care, streamline operations, and reduce costs. On the other, strict privacy regulations, limited budgets, and a “first, do no harm” culture create a seemingly impenetrable web of constraints. Yet constraints need not be barriers; they can serve as catalysts for more imaginative, robust, and trusted AI implementations.
This post offers practical strategies for CTOs navigating Australia's public and private healthcare sectors. We'll also peer into the near-future of AI, especially agent-based systems, and examine how constraints can guide us toward safer, stronger innovation.
The Australian Healthcare AI Dilemma
In many ways, Australia's healthcare system epitomizes the tension between AI's potential and the barriers of real-world adoption. Privacy and safety considerations loom large, with the Privacy Act, state-specific health data rules, and the Therapeutic Goods Administration (TGA) regulating AI used for medical diagnoses or interventions. Culturally, clinicians are understandably cautious, preferring proven efficacy over hype. Meanwhile, tight budgets require solid proof of ROI before a project gets green-lit.
Paradoxically, AI research is prolific, but live deployments remain sparse. Many pilot projects struggle to leave the sandbox phase, and clinician trust can be slow to build. Successful innovation must therefore embrace these constraints, embedding regulatory compliance, data safeguards, and clinical collaboration from the very start.
1. Start Small with Low-Risk, High-Impact Pilots
Why Pilots Matter
In a cautious industry, pilots are a way to prove AI's value on a controlled stage. By focusing on specific workflows, like automating administrative tasks or offering decision support in low-stakes scenarios, CTOs can gather evidence of success with minimal risk. These early wins reduce fear of the unknown and build a trustworthy track record for AI.
Key Tactics
- Problem-Driven Approach: Partner with clinicians to identify a real pain point that AI can address in a contained environment.
- Early Governance Engagement: Loop in ethics committees, legal, and clinical leadership from day one, ensuring compliance is built into the pilot.
- Measure & Broadcast Success: Whether you reduce a manual workflow by 20% or improve timely interventions, highlight the benefits to establish credibility and buy-in.
2. Harness Synthetic Data to Bypass Privacy Roadblocks
Why Synthetic Data?
Healthcare data is heavily regulated, and for good reason: patient confidentiality is sacrosanct. Synthetic data, artificially generated datasets that mirror the statistical properties of real patient data, offers a low-risk sandbox for AI model development. Developers can experiment freely without exposing personal information.
How It Drives Innovation
- Rapid Model Iteration: Acquire, share, and refine models without months of approvals for real data usage.
- Collaboration Made Easier: Synthetic datasets can be legally shared with vendors or research partners.
- Edge Cases & Rare Conditions: You can oversample uncommon clinical scenarios, making models more robust.
Cautionary Note
Poorly generated synthetic data can embed bias or fail to replicate real-world edge cases. Rigorous validation on a de-identified real dataset is essential to ensure performance.
3. Cultivate a Culture of Compliance-Aware Innovation
Balancing Creativity with Caution
Healthcare organizations often default to a conservative stance: “Wait and see if AI really works somewhere else first.” To make steady progress, leaders must model a mindset in which controlled risk-taking is not just allowed but encouraged, provided it's done in a deeply compliance-focused way.
Practical Steps
- Multidisciplinary “AI Councils”: Involve privacy officers, clinicians, data scientists, and legal from the outset to review proposals. This ensures problems are caught early and fosters a shared sense of responsibility.
- Training & Communication: Teach staff why compliance matters and highlight how good governance protects both patients and innovators.
- Human-Centered Design: Present AI as an enhancement, not a replacement. Show how automation can free staff for higher-value tasks, avoiding the impression that robots are here to replace clinicians.
4. The Rise of Agent-Based AI Systems
Agent-based AI systems represent a new frontier. Unlike traditional models that offer a static output, agents can act on information, coordinating tasks, interacting with environments, and collaborating with other agents or systems.
What This Looks Like
- Autonomous Orchestration: An AI agent flags a high-risk patient, then schedules follow-up appointments, alerts care teams, and maintains an ongoing monitoring loop.
- Multi-Agent Ecosystems: Different agents handle specialized roles, e.g., data synthesis, care-plan management, and communicate for holistic patient oversight.
Early Use Cases
- Discharge Management: Automating the labyrinth of post-discharge tasks (scheduling GP visits, ordering home-care resources, setting reminders).
- Administrative Workflows: Checking insurance claims or triage tasks, streamlining processes and freeing clinicians to focus on care.
5. Innovating in a Wait-and-Watch Landscape
Australia's healthcare boards often adopt a conservative “wait-and-watch” posture. Savvy CTOs work within this cultural norm, introducing carefully staged innovations that, over time, expand the organization's appetite for AI.
Incremental Wins Over Moonshots
- Prove Value Quickly: Start by shaving 10% off a time-consuming workflow rather than proposing to automate an entire hospital ward.
- Regulatory Sandboxes: Lobby for or participate in test environments where new AI tools can be tried under strict oversight but with less red tape.
- Evidence & Empathy: Show local stakeholders how AI was adopted safely elsewhere, and ground every big-picture vision in concrete data.
6. Lessons from Global Healthcare Innovators
No system has fully mastered AI adoption yet, but global examples can inform Australian efforts:
- United Kingdom (NHS AI Lab): A government-backed collaboration unites regulators, clinicians, and tech firms, funding AI through structured, regulated pilots.
- United States (Duke Health Sepsis Watch): Gradual rollouts with human-in-the-loop oversight built trust and delivered measurable gains in early sepsis detection.
- Israel & Singapore: Smaller nations with strict privacy laws use carefully governed data lakes and regulatory sandboxes to accelerate experimentation without compromising patient safety.
Common Thread: Success stories emphasize measured pilot projects, human-centric design, stakeholder collaboration, and carefully built data infrastructure.
7. Future-Proofing for the Next 3-5 Years
Large Language Models (LLMs)
Expect more specialized medical LLMs capable of summarizing records and providing real-time clinical assistance, all behind secure firewalls. CTOs should prepare for easy integration (e.g., APIs, middleware) and the demands of explainability.
Predictive to Prescriptive
AI that recommends interventions and autonomously initiates low-risk follow-up actions will become more common, requiring robust workflows and well-defined boundaries for autonomous decision-making.
Personalized Medicine & Genomics
As genomic sequencing gets cheaper, AI will be critical in tailoring treatments to individual genetic profiles. Healthcare IT must be ready to handle and secure this sensitive data.
Evolving Regulation
Australia is drafting new frameworks on “Safe and Responsible AI in Health Care.” Building compliance and audit trails into current systems will make future adoption easier and help avoid retrofitting solutions later.
Conclusion: The Constraint-Savvy Leader
Leading AI innovation in Australian healthcare will always be a careful dance between bold creativity and unwavering caution. The constraints are real, budgetary pressures, regulatory scrutiny, and cultural skepticism, but they need not be showstoppers.
By:
- Starting small with impactful pilots,
- Leveraging synthetic data to tackle privacy concerns,
- Ingraining a culture of compliance-aware innovation, and
- Keeping an eye on the agent-based AI horizon,
CTOs can transform constraints into guardrails that guide them toward safer, smarter solutions. It is in these carefully orchestrated, iterative steps that AI's promise truly emerges: a world where clinicians remain firmly in control but benefit from tireless, intelligent assistants. The best leaders will recognize that constraints, far from hampering progress, can actually illuminate the path to robust, trusted AI adoption.
References
-
Artificial intelligence 'facing barriers' in our health system | InSight+
https://insightplus.mja.com.au/2024/1/artificial-intelligence-facing-barriers-in-our-health-system -
Artificial Intelligence (AI) and medical device software | Therapeutic Goods Administration (TGA)
https://www.tga.gov.au/how-we-regulate/manufacturing/manufacture-medical-device/manufacture-specific-types-medical-devices/artificial-intelligence-ai-and-medical-device-software -
Hospitals Roll Out AI Systems to Keep Patients From Dying of Sepsis - IEEE Spectrum
https://spectrum.ieee.org/hospitals-roll-out-ai-systems-to-keep-patients-from-dying-of-sepsis -
New report reveals the reality of AI adoption in London healthcare - UCLPartners
https://uclpartners.com/news-item/new-report-reveals-the-reality-of-ai-adoption-in-london-healthcare -
Synthetic Data in Healthcare: the Great Data Unlock - Hospitalogy
https://hospitalogy.com/articles/2023-11-02/synthetic-data-in-healthcare-great-data-unlock -
Adaptability and promising potential of synthetic information in healthcare
https://www.news-medical.net/news/20231011/Adaptability-and-promising-potential-of-synthetic-information-in-healthcare.aspx -
Artificial intelligence in healthcare: transforming the practice of medicine - PMC
https://www.ncbi.nlm.nih.gov/pmc/articles/PMC8285156 -
Towards Next-Generation Medical Agent: How o1 is Reshaping Decision-Making in Medical Scenarios
https://arxiv.org/abs/2411.14461 -
Demystifying Agentic AI: How AI Agents Can Change Healthcare Efficiency Now - Productive Edge
https://www.productiveedge.com/blog/demystifying-agentic-ai-how-ai-agents-can-change-healthcare-efficiency-now -
The NHS AI Lab - NHS Transformation Directorate
https://transform.england.nhs.uk/ai-lab -
Consultation on Safe and Responsible AI in Health Care - Australian Department of Health and Aged Care
https://consultations.health.gov.au/medicare-benefits-and-digital-health-division/safe-and-responsible-artificial-intelligence-in-he