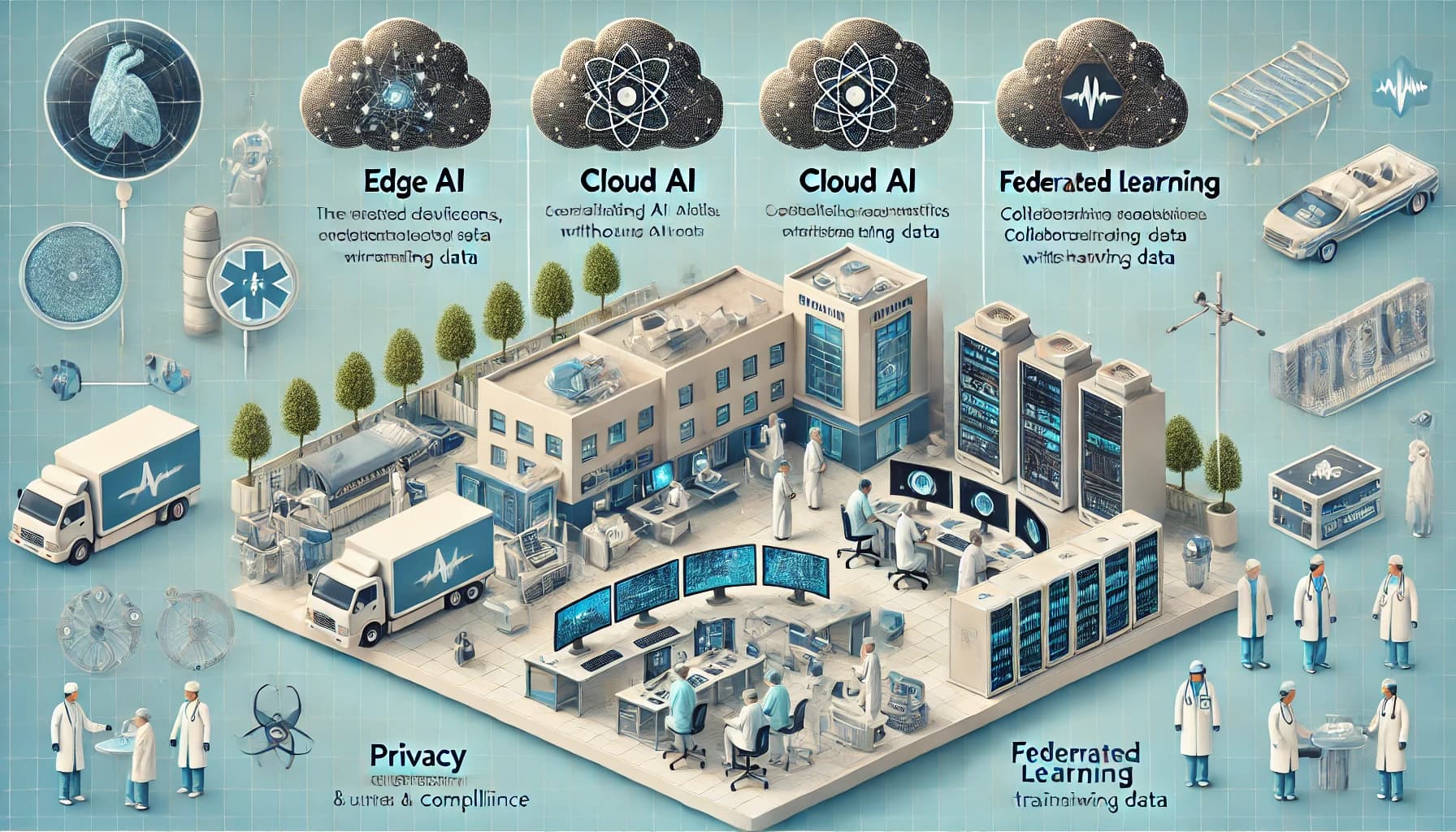
Emerging AI Architectures in Australian Healthcare: Edge, Cloud, and Federated Learning
Australia's healthcare system is on the cusp of an AI-driven transformation. Leaders face a strategic question: how do we harness AI's power for immediate clinical decisions and long-term population insights, while navigating strict privacy laws and the demands of real-world care? The answer lies in combining emerging AI architecture patterns—edge AI, cloud-based AI, and federated learning—each addressing key needs like latency, privacy, and compliance in unique ways. In this thought leadership exploration, we'll dive into forward-looking trends for each architecture, their trade-offs and benefits, and how hybrid models blending all three could define the future of Australian healthcare.
The Need for Low Latency and High Privacy in Healthcare AI
In medicine, every second counts. Whether it's an AI flagging a critical abnormality on an X-ray or monitoring a patient's vitals, latency (the speed of AI decisions) can mean life or death. Equally important is privacy—patient data is highly sensitive, and Australia's regulations (from the Privacy Act to the My Health Records Act) demand careful stewardship. In fact, under the My Health Records Act, all data in the national My Health Record system must be stored on Australian soil (Australian data sovereignty guide for multinational companies - InCountry), reflecting the priority placed on data sovereignty and compliance. Traditional approaches of centralizing all health data are proving impractical in such an environment. Australian experts now recognize that “complete centralisation in healthcare was always an unrealistic goal”, given how diverse and dispersed health data is (The AI Revolution is Transforming Data Management in Australian Healthcare). This realization is driving interest in federated and distributed approaches that securely access or learn from data where it resides (The AI Revolution is Transforming Data Management in Australian Healthcare).
Against this backdrop, three AI architecture patterns have emerged as particularly promising:
- Edge AI - AI that runs on-site (on hospital premises or on devices) for real-time, local decision-making.
- Cloud-based AI - AI that leverages centralized cloud infrastructure for heavy analytics, scalable training, and cross-institution insights.
- Federated Learning - AI model training method that enables collaboration across institutions without sharing raw data, keeping data local and only exchanging learned model parameters.
Each pattern offers distinct strengths for Australian healthcare, from ultra-fast bedside decisions to secure multi-hospital collaboration. Let's examine each in turn, and then see how combining them can yield a best-of-all-worlds solution.
Edge AI: Intelligence at the Bedside and in the Field
Edge AI brings the power of artificial intelligence directly to the point of care - running on local devices like medical scanners, monitors, or even mobile kits - rather than in a distant data center. By processing data on-site, edge AI delivers ultra-low latency insights, which is invaluable in clinical scenarios where instant decisions save lives. For example, GE Healthcare has embedded an AI algorithm on its X-ray machines to detect critical findings in under one second, flagging life-threatening cases for immediate attention (Intel and GE Healthcare Partner to Advance AI in Medical Imaging). This on-device model (optimized with tools like Intel's OpenVINO) slashed the X-ray analysis time from over 3 seconds to less than 1 (Intel and GE Healthcare Partner to Advance AI in Medical Imaging) - a dramatic improvement that helps radiologists prioritize emergencies without waiting for cloud processing.
Enhanced privacy is another hallmark of edge AI. Patient data processed at the edge doesn't need to leave the hospital or device, reducing exposure risks. In an Australian context, this is a boon. It aligns with stringent privacy principles and keeps data under local control (important when laws discourage sending health information offshore (Australian data sovereignty guide for multinational companies - InCountry)). Consider remote healthcare in Western Australia: a newly developed “Medi-Kit” deployed on 4WD vehicles brings diagnostics to remote Aboriginal communities, equipped with onboard ECG, ultrasound, and cameras using edge AI analytics right on the device (The Challenge - Healthy Connections). This kit can screen for heart and chronic diseases on-site, supported only by satellite connectivity for occasional updates (The Challenge - Healthy Connections). By analyzing data locally (even in the middle of the Pilbara), patients get immediate results and better continuity of care, without raw data streaming over fragile networks. Edge AI's ability to function with limited or intermittent connectivity makes it a lifeline for rural and remote Australian regions where network latency or outages are common.
That said, trade-offs exist. Edge devices have constrained computing resources and energy. AI models must often be compressed or optimized to run efficiently - which can slightly limit complexity or accuracy compared to massive cloud servers. There's also the challenge of maintaining and updating many distributed models: a hospital might have hundreds of edge AI-enabled devices (from bedside monitors to MRI machines) that all need the latest knowledge. Despite these challenges, the benefits are compelling:
- Real-time decision support: Critical alerts and analyses happen instantaneously at the bedside or in the field, improving acute care response times.
- Data stays local: Sensitive information is processed on-site, boosting patient trust and easing compliance since less data leaves the premises (Australian data sovereignty guide for multinational companies - InCountry).
- Resilience to connectivity issues: Clinical AI tools remain operational even if internet connections fail - crucial for both urban hospitals (during outages) and remote clinics on satellite links.
- Device innovation: Companies are rising to the challenge of edge computing constraints. NVIDIA's Clara platform, for instance, offers an embedded AI toolkit (Clara AGX) that can be built into medical instruments for high-rate image and video processing (iTWire - Nvidia federates healthcare ML training). One early example is the Hyperfine portable MRI - a small, wheeled MRI machine using on-board AI to assist imaging in places traditional MRIs can't go (iTWire - Nvidia federates healthcare ML training). These innovations show how edge AI is not only feasible but already transforming care delivery at the device level.
For Australian healthcare providers, adopting edge AI can mean an ICU patient's monitor that immediately detects an arrhythmia, or a point-of-care diagnostic that gives answers within a GP visit. It brings the intelligence of AI into the flow of care without waiting on distant servers - all while keeping patients' data close, secure, and private.
Cloud-Based AI: Scalable Intelligence and Big-Picture Insights
In contrast to the localized focus of edge computing, cloud-based AI taps into virtually unlimited computing power and storage in centralized data centers. The cloud is where health data from across cities, states, or the entire country can converge (with proper consent and security) to fuel advanced analytics and model training at scale. Scalability is the key word: training a cutting-edge diagnostic model might require crunching millions of records or imaging scans - a task suited for a cloud GPU cluster or high-performance server farm, not an on-premises PC. Cloud AI enables healthcare organizations to build more complex, accurate models by pooling larger datasets than any single hospital could alone.
Australia's healthcare leaders are increasingly leveraging cloud platforms for this very reason. A recent example is Ramsay Health Care (one of the nation's largest private hospital networks) partnering with Google Cloud to create a centralized “data hub” spanning its 70+ facilities (Ramsay Health Care scaling AI adoption with Google Cloud | Healthcare IT News) (Ramsay Health Care scaling AI adoption with Google Cloud | Healthcare IT News). This hub, built on Google's BigQuery data warehouse, will ingest and unify real-time data from formerly siloed systems and serve as a single source of truth (Ramsay Health Care scaling AI adoption with Google Cloud | Healthcare IT News). The immediate goal is to break down data silos to enable insights-driven care - Ramsay noted that having data locked in separate on-premise systems made analysis “time-intensive and cumbersome,” so a cloud consolidation will “operationalise data, improve connectivity, and unlock new insights to support clinical decision-making.” (Ramsay Health Care scaling AI adoption with Google Cloud | Healthcare IT News) In practice, that means patterns in patient outcomes or operational inefficiencies that were previously hidden can be discovered through cloud-based analytics. The cloud hub also lets Ramsay deploy AI and ML tools at scale, applying algorithms to everything from predicting patient deterioration to optimizing hospital workflows (Ramsay Health Care scaling AI adoption with Google Cloud | Healthcare IT News).
Advanced analytics and longitudinal insights are major benefits of cloud AI. Because data from many sources can be aggregated, healthcare providers can analyze trends over years and across populations. Public health agencies could use cloud-based AI to model disease outbreaks or assess the efficacy of interventions by drawing on data from across Australia. Likewise, researchers can collaborate on de-identified national datasets to uncover, say, early indicators of chronic illness—insights that no single clinic's data would reveal.
However, with great power comes great responsibility (and a few drawbacks). Network dependency is one: cloud AI assumes reliable connectivity. If a hospital's link to the cloud is slow or drops out, real-time AI services may be disrupted. This isn't a trivial concern - in critical care, a few minutes of network downtime shouldn't halt decision support. That's why many hospitals use cloud AI for analysis that isn't ultra-time-sensitive, or they pair it with edge solutions for the “last mile” immediate response. Another consideration is regulatory compliance around data transfer and storage. Uploading patient data to the cloud must be done in line with Australia's privacy laws and guidelines. Healthcare data often must remain within Australian jurisdiction; notably, the My Health Record system is legally required to be stored on servers within Australia (Australian data sovereignty guide for multinational companies - InCountry). Cloud providers have responded by offering local data centers and compliance certifications. In Ramsay's case, all patient data in the Google Cloud hub is encrypted end-to-end (Ramsay Health Care scaling AI adoption with Google Cloud | Healthcare IT News), and presumably stored in Australian regions to meet data sovereignty needs.
Key advantages of cloud-based AI in healthcare include:
- Massive scale for training and analytics: Complex models (e.g. detecting subtle genetic risk factors or rare diseases) can be trained on tens of thousands of records in the cloud, far beyond what an edge device could handle. This leads to more accurate and generalizable AI tools.
- Centralized model refinement: A cloud setup allows continuous learning - models can be updated as new data streams in from many clinics, ensuring the AI's knowledge stays up-to-date. For example, if a new strain of virus emerges, a central model can learn from cases nationwide and quickly disseminate updated insights.
- Population-level insights: Cloud platforms can analyze aggregated, de-identified data for epidemiological trends, resource planning, and research. This can inform policy and proactive care. (Imagine a national model predicting hospital bed demand during flu season by analyzing trends from every region.)
- Easier multi-site integration: Through cloud APIs and interoperability solutions, data from different hospital IT systems can be harmonized. In fact, Ramsay's cloud project uses Google's Apigee and Anthos to ensure different systems talk to the central hub securely (Ramsay Health Care scaling AI adoption with Google Cloud | Healthcare IT News). This kind of integration is a stepping stone to the “single digital health record” vision Australia has long pursued - except now it can be virtual via cloud rather than one monolithic database.
Of course, mitigating the downsides is part of the strategy. Ensuring low latency access might mean using hybrid cloud setups or edge preprocessing (more on that soon). And strict governance, encryption, and audit trails in the cloud are a must to maintain patient trust. Fortunately, cloud providers are well aware: major platforms come with compliance attestations (HIPAA, GDPR, and local standards) and tools for data de-identification and loss prevention (What is the Google Cloud Healthcare API? - Paubox) (What is the difference between Google Cloud Healthcare API vs ...). The Australian government itself has begun trusting public cloud for health data - the Federal Department of Health and Aged Care recently tapped Google Cloud to help consolidate its data assets securely (DoHAC appoints Google to consolidate data assets), a strong signal that with the right safeguards, cloud AI is part of Australia's health future.
Federated Learning: Collaboration Without Data Sharing
While cloud AI centralizes data for collective insight, Federated Learning (FL) takes a radically different approach: keep the data distributed, bring the algorithms to the data. In federated learning, each hospital or device trains the AI model locally on its own data, then shares only the learned model updates (parameters) with a central coordinator (or shares with each other in a peer-to-peer manner). The central server (or federation mechanism) then aggregates these updates to form a improved global model, which is sent back out to all participants. At no point are raw patient records or images pooled centrally - they stay in their original database or device. This technique was pioneered in part to deal with privacy and regulatory hurdles that limit data sharing (What's Federated Learning and why it's key to the future of medical AI | by Rachel Dulberg | Medium) (What's Federated Learning and why it's key to the future of medical AI | by Rachel Dulberg | Medium). It's increasingly viewed as “key to the future of medical AI” because it offers a path to build robust, accurate models without violating data privacy (What's Federated Learning and why it's key to the future of medical AI | by Rachel Dulberg | Medium).
In the Australian healthcare context, federated learning could be transformative. Consider the challenge of building an AI model to detect a rare cancer: no single hospital has enough cases to train a reliable algorithm. Traditionally, one might try to gather all data into a central repository - a process fraught with privacy red tape and public concern. With FL, however, major hospitals in Sydney, Melbourne, Perth, and Brisbane could collaborate on training a shared model without ever exchanging patient records directly. Each site's data stays on-premises, satisfying local compliance, while the collective knowledge is achieved through parameter sharing. This approach inherently respects data sovereignty (each hospital controls its data). It's also well-aligned with Australia's Privacy Principles that emphasize minimizing use and disclosure of personal info. In effect, federated learning makes privacy a feature, not just an afterthought.
The benefits go beyond just appeasing regulations. Federated models tend to be more generalizable: because they learn from a diversity of data sources (different demographics, equipment, clinical protocols across sites), the resulting AI can perform more robustly when deployed broadly. For example, a federated learning project in the UK enabled a dozen NHS hospitals to jointly train a model for detecting brain tumors on MRI scans (iTWire - Nvidia federates healthcare ML training). None of the hospitals saw each other's images, yet the combined model was more accurate for each of them than any could have achieved alone. NVIDIA's Clara Federated Learning platform facilitated this by allowing each hospital to train on its own, then share model weights to build a global model (iTWire - Nvidia federates healthcare ML training) (iTWire - Nvidia federates healthcare ML training). Similar efforts have been piloted in the U.S. (by the American College of Radiology) and are catching interest worldwide as a way to break down “data silos” in healthcare while preserving patient confidentiality (iTWire - Nvidia federates healthcare ML training). It's easy to envision Australian institutions following suit - for instance, state health departments or research collaborations using FL to train AI on pathology images or genomic data spread across the country's top labs.
However, federated learning is not without challenges and trade-offs:
- Complexity and Infrastructure: FL requires coordination - a central server to aggregate models, or a peer-to-peer scheme, along with secure communication channels. Each participating site needs sufficient compute power to train models locally (which might mean investing in GPU servers or high-end edge devices at each hospital). There's also the need for synchronization (all sites perhaps need to train on the latest model epoch at roughly the same time) and robust monitoring of the training process. This can be complex to set up and maintain, especially across independent organizations.
- Privacy isn't absolute: While raw data isn't shared, the model updates themselves can, in theory, leak some information if intercepted or if a malicious participant tries to reverse-engineer data from them (Privacy-Enhanced Federated Learning - Privacy Technology Research Group). Researchers (including CSIRO's Data61 team (Privacy-Enhanced Federated Learning - Privacy Technology Research Group)) are actively working on privacy-enhancing techniques like differential privacy and secure multiparty computation to harden federated learning against such risks. For now, federated learning greatly reduces privacy risk but doesn't eliminate it entirely - health CTOs should still encrypt model parameters in transit and possibly randomize them to prevent reconstruction of sensitive details (Privacy-Enhanced Federated Learning - Privacy Technology Research Group).
- Convergence and validation: Training in a distributed way can sometimes converge more slowly or be tricky to validate. If one hospital's data is very different (say one pathology lab has much older staining techniques than others), it might throw off the global model if not handled (techniques like weighting updates or doing a few central rounds can help). Ensuring the final model works well for everyone and is clinically validated requires careful testing - ideally each participant validates the model on their local test data.
Despite these challenges, federated learning's potential in Australia is immense. It essentially enables a “federated health data system”, where instead of aggregating data in one place, we securely access or learn from data in place (The AI Revolution is Transforming Data Management in Australian Healthcare). This approach is “more practical — essential for providing comprehensive, timely care in the age of AI” as one Australian health data expert noted (The AI Revolution is Transforming Data Management in Australian Healthcare). By embracing FL, Australian healthcare can tap into nationwide (even international) AI developments while sidestepping many legal pitfalls. We could see, for example, a coalition of major teaching hospitals training a shared AI for stroke detection on brain scans; each hospital keeps its scans on-site, yet benefits from a model trained on a volume of data collectively much larger than any single institution holds. The generalizability of such a model would be a big win - likely able to handle patient variability across Australia's multicultural population better than siloed models.
Hybrid Models: Combining Edge, Cloud, and Federated Approaches for Maximum Impact
The real power of these architectures emerges when we combine them. The future of AI in Australian healthcare isn't about choosing edge versus cloud versus federated - it's about orchestrating all three in a complementary fashion. By doing so, healthcare organizations can deliver both immediate, bedside intelligence and broader, population-level knowledge building. Let's paint a picture of how a hybrid model might work in practice:
-
At the Edge (Clinical Frontlines): A patient in a rural NSW clinic undergoes an ultrasound. The device is equipped with an edge AI model (let's say for detecting signs of kidney disease). The analysis happens on-device in seconds, giving the GP an instant preliminary report. Because this inference is local, it works even with the clinic's spotty internet and the patient's data stays in the exam room (addressing privacy). If the AI finds something urgent, it flags it immediately so that care can be expedited or a specialist consult arranged.
-
In the Cloud (Central Brain): Later, an anonymized summary of that ultrasound finding (or the image, if policy allows) is uploaded to the health service's secure cloud database. There, it joins thousands of other records from clinics and hospitals across the region. In the cloud, a more comprehensive analysis might occur: for instance, aggregating data to see if this region is seeing an unusual uptick in kidney issues, or to refine risk prediction models by incorporating this new data point. The cloud also hosts a more complex AI model that was trained on a vast dataset; periodically, this model is updated and can send improvements back to the edge devices. In our scenario, the cloud might crunch data overnight and determine a better way to identify early kidney disease, creating a new version of the model.
-
Federated Learning (Collaboration Backbone): Suppose multiple healthcare networks - in NSW, Queensland, Victoria - agree to collaborate to improve AI models for a range of conditions. Instead of pooling all data into one giant repository (which could raise cross-border data sharing issues and political hurdles), they use federated learning. Each network's cloud system (or major hospital's server) trains on its local data (which includes contributions from their edge devices). They then securely share the learned model updates to a central federation server that aggregates a national model. This federated model captures patterns from, say, both metropolitan tertiary hospitals and small rural clinics, making it widely applicable. The national model is then sent back to each participant's cloud, and from there, deployed out to their edge devices (the ultrasound machines, the X-ray devices, the EHR decision support at bedside, etc.). In this way, the collective intelligence of the whole country's healthcare system updates the local intelligence at each point of care. Immediate clinical decisions benefit from the latest global insights, and conversely, local outcomes continuously inform the global knowledge.
Such a hybrid architecture takes the strengths of each approach and mitigates their weaknesses. The edge provides the speed and privacy for frontline care, the cloud provides the heavy lifting for training and aggregated insight, and federated learning provides the conduit for collaboration without breaching privacy boundaries. We're essentially establishing a virtuous cycle: edge devices generate data and deliver care, cloud systems learn from the data (in aggregate) to improve models, and federated learning allows multiple clouds or edge networks to share in those improvements without sharing raw data.
We can see early signs of this convergence. NVIDIA's aforementioned Clara platform, for example, runs its federated learning on the NVIDIA EGX edge computing platform (iTWire - Nvidia federates healthcare ML training) - effectively bringing the FL process to where the data lives (at hospital edge servers), and then only syncing model weights. This kind of edge-cloud blur means a hospital might have a mini-cloud on-premises (for speed and privacy) that still connects to a bigger cloud or federation for collective learning. Likewise, Google Cloud's healthcare offerings and others are increasingly supporting hybrid deployments (with tools like Anthos or Azure Stack) so that hospitals can keep certain sensitive workloads on-site while still benefiting from cloud-scale analytics. In Australia, where data sovereignty is a top concern, such hybrid cloud setups are appealing: they allow compliance with “data must stay in-country/in-house” requirements while leveraging cloud innovations.
Latency vs. Insight Trade-off - solved: By combining edge and cloud, hospitals don't have to choose between instant responsiveness and rich analytical insight. Edge handles the former; cloud handles the latter. A cardiology department might use edge AI to monitor patients' heart rhythms in real-time for arrhythmias, but all those rhythms can be pooled (with consent) in the cloud to discover longer-term patterns (e.g., predicting an oncoming heart condition weeks in advance). They get the acute alerts and the chronic trend analysis in one system.
Privacy vs. Collaboration - solved: Federated learning ensures that participating in a nation-wide (or even international) AI initiative doesn't mean handing over your patients' identities. Each provider can contribute to, say, a new drug efficacy AI model without uploading any confidential data - a huge win for compliance with laws like the Privacy Act and institutional policies. The collaboration happens in the model parameter space, not the data itself, preserving data locality and patient confidentiality by design.
In summary, a hybrid edge-cloud-federated model aligns perfectly with the dual objectives of modern healthcare: optimizing individual patient outcomes in the moment, and continuously learning to improve outcomes for all patients over time. It's a way to have our cake and eat it too - ultra-fast, local AI and broad, learning AI. For Australian healthcare CTOs and innovation leaders, the strategic direction is clear: embrace a layered AI architecture.
Strategic Outlook: Towards an AI-Augmented Healthcare Ecosystem
As we look to the future of AI in Australian healthcare, it's evident that no single architecture will dominate. Instead, the leading healthcare organisations are crafting AI ecosystems that leverage edge, cloud, and federated learning where each fits best. The National Digital Health Strategy (2023-2028) signals a push for connected, data-rich infrastructure that delivers information “anywhere, anytime” while upholding privacy and security (Australian Digital Health Agency seeks partners for infrastructure transformation - Services - CRN Australia) - a vision practically unattainable without a blend of edge computing (for anytime access on-site) and cloud/federation (for connecting across settings with consent). Innovation is happening on all fronts: from Australian startups like Harrison.ai developing advanced clinical AI models (often trained in the cloud on vast datasets) to federal initiatives ensuring those models can be safely deployed in practice. We see global signals influencing the local market too. Europe's GDPR accelerated interest in federated learning - similarly, Australia's strong privacy stance makes FL attractive as a standard practice for multi-institutional AI research. Major tech players are tailoring their healthcare platforms to Australia's needs (as with Google Cloud's encryption and local hosting for Ramsay (Ramsay Health Care scaling AI adoption with Google Cloud | Healthcare IT News), or Microsoft supporting projects like DrumBeat.ai that use cloud AI to reach remote Indigenous communities (DrumBeat.ai project taps AI and cloud to tackle Indigenous hearing challenge - Microsoft Australia News Centre)).
For healthcare CTOs, the path to AI-driven transformation involves balancing immediacy and insight:
- Leverage Edge for Critical Workloads: Identify where low latency truly matters - e.g., emergency diagnostics, surgery assistance, bedside monitoring - and invest in edge AI solutions there. Whether it's smart vital sign monitors or AI-guided imaging devices, these give clinicians “instant AI” that feels like having an expert always on hand. Australian hospitals might deploy edge AI in ambulances or rural clinics to extend specialist capabilities to the frontlines.
- Use Cloud for Unified Data and Innovation: Continue consolidating and cleaning data in secure cloud environments to enable the magic of big-data AI. The cloud should become your “AI innovation factory” - a place to experiment with new models, run heavy analytics, and generate the insights that feed back into practice. Ensure your cloud strategy aligns with local compliance: choose in-country data centers (Australian data sovereignty guide for multinational companies - InCountry) and use privacy-preserving tools (de-identification, encryption) so that even as you aggregate data, you maintain public trust and legal integrity.
- Pilot Federated Learning for Collaboration: Start with pilot projects in federated learning, perhaps via partnerships with other hospitals or research institutes. Good candidates are areas where data sharing is sensitive but collaboration is beneficial - for example, training AI on rare diseases, or multi-site drug trial data analysis. By getting comfortable with FL frameworks (there are open-source options and offerings from NVIDIA, Intel, and others), your organization will be ready for a future where FL could underpin nationwide AI efforts (imagine if each state's health system contributes to a national AI for cancer care, all without centralizing data).
- Architect for Hybrid: Design your systems with a hybrid mindset. This might mean deploying containerized AI models that can run on-premises or in cloud seamlessly, ensuring interoperability between edge devices and cloud services, and adopting standards (like FHIR for health data) that make it easier to exchange information securely. A patient's data should flow from device to cloud to federated network as needed, invisibly and securely, to deliver value at each stop. The technical implementation might be complex, but the end experience for clinicians and patients will be a smoothly augmented healthcare journey.
The future potential is exhilarating. We could see an Australia where an ICU in Melbourne uses edge AI to manage ventilators with minute-by-minute precision, while simultaneously contributing anonymized data to a cloud AI system tracking a national respiratory illness trend, which in turn is being refined by federated learning from ICUs in Sydney and Brisbane - a full loop of continuous learning and improvement. Latency, privacy, and compliance challenges can be tackled by this layered approach: immediate needs handled on the edge, big-picture needs handled in the cloud, and collaboration facilitated by federated techniques that respect boundaries.
In conclusion, embracing edge AI, cloud AI, and federated learning in harmony offers Australian healthcare a path to smarter, faster, and safer care. It's about putting AI where it needs to be - at the bedside when speed is vital, in the cloud when scale is needed, and in the federated network when sharing is required but privacy must hold. By investing in these emerging architecture patterns and, crucially, knitting them together, healthcare providers can achieve a powerful synergy: delivering world-class immediate clinical decisions and building longitudinal insights that improve outcomes for all Australians. The technology and frameworks are largely here - from NVIDIA Clara to Google Cloud's Healthcare tools to initiatives born on our own shores - and the coming years will be about implementation and innovation. For healthcare CTOs and leaders, the mandate is clear: think holistically, plan for hybrid AI, and lead the charge in turning these forward-looking trends into everyday healthcare reality.
Sources:
- Australian data sovereignty and privacy requirements for health data (Australian data sovereignty guide for multinational companies - InCountry)
- NVIDIA Clara Federated Learning - enabling multi-hospital AI model training without sharing patient data (iTWire - Nvidia federates healthcare ML training)
- NVIDIA Clara, EGX platform and embedded AI in medical devices (e.g. Hyperfine portable MRI) (iTWire - Nvidia federates healthcare ML training)
- GE Healthcare's edge AI on X-ray devices using Intel OpenVINO (sub-second critical condition detection) (Intel and GE Healthcare Partner to Advance AI in Medical Imaging)
- Western Australia “Medi-Kit” with edge AI for remote community healthcare (Curtin Univ. project) (The Challenge - Healthy Connections)
- Ramsay Health Care's Google Cloud data hub for unified analytics and AI in Australia (Ramsay Health Care scaling AI adoption with Google Cloud | Healthcare IT News) (Ramsay Health Care scaling AI adoption with Google Cloud | Healthcare IT News)
- DrumBeat.ai remote ear health project using cloud AI to assist rural care via telehealth (DrumBeat.ai project taps AI and cloud to tackle Indigenous hearing challenge - Microsoft Australia News Centre)
- Commentary on federated health data systems gaining traction in Australia (decentralized data access) (The AI Revolution is Transforming Data Management in Australian Healthcare) (The AI Revolution is Transforming Data Management in Australian Healthcare)
- CSIRO Data61 on privacy considerations in federated learning (risks of data leakage from model updates) (Privacy-Enhanced Federated Learning)