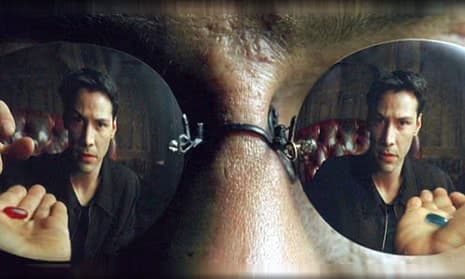
Avoiding GenAI Vendor Lock-In: Embracing Open, Containerized ML Pipelines
Introduction: A Cautious Approach to the Generative AI Boom
We find ourselves in a phase of breakneck AI innovation, particularly in the realm of large language models (LLMs) and generative AI. Yet along with all this promise looms a familiar specter: vendor lock-in. Being tethered too tightly to a single AI or cloud provider can hamstring agility, bloat costs, and ultimately stifle innovation.
Forward-looking organizations are therefore seeking open, containerized ML pipelines: flexible, portable, and standards-based solutions that confer the freedom to experiment, the power to optimize, and the ability to switch platforms if (or when) circumstances demand. Let us step through the implications of vendor lock-in in the generative AI era, and how an open, containerized approach can save you from the swirling gravitational pull of proprietary traps.
The Vendor Lock-In Conundrum in ML and Generative AI
Lock-in is hardly new, but in the fast-moving world of ML and generative AI, the stakes are magnified:
- Limited Flexibility
Betting on a single closed platform leaves you at the mercy of that vendor's roadmap, pace of innovation, and pricing. If newer, better models or solutions emerge, migrating can be laborious or downright impossible. - Higher Costs & Weakened Negotiation
Once a vendor has you captive, unexpected price hikes can erode your AI budget. Multi-year deals can likewise prove disastrous if a more cost-efficient alternative arises. - Operational Risk
Relying on a third party's closed service can backfire during outages or abrupt organizational changes within the vendor itself (we all remember the OpenAI leadership saga). - Data & Compliance Hurdles
When your proprietary data is locked in a vendor's environment or format, multi-cloud flexibility, regulatory compliance, and control over your AI systems become exponentially more complex.
The overarching theme? Architect for optionality. Set yourself up to deploy on any cloud, swap in new models, and avoid dependency on a single point of failure. The path forward, as many large enterprises have discovered, is the strategic adoption of open, containerized workflows.
Why Open, Containerized Workflows Are the Future
1. Portability: “Build Once, Run Anywhere”
Containers package your entire ML application, dependencies and all, so it can be dropped onto any platform (on-prem, AWS, Azure, GCP, or the edge) with minimal friction. With Kubernetes as the ubiquitous container orchestrator, you can migrate or split workloads across multiple infrastructures and avoid painting yourself into a corner.
2. Scalability and Flexibility on Demand
Scalable orchestration frameworks (like Kubernetes + Ray) enable dynamic resource allocation and graceful autoscaling. Your containerized ML microservices can run on a single GPU or sprawl across a massive compute cluster, without tying you to a vendor's specialized service.
3. Cost Control and Efficiency
Open containerized solutions let you optimize infrastructure usage (often cutting GPU overhead dramatically) and circumvent the costly egress fees or usage-based pricing surprises imposed by some AI vendors. You're free to mix-and-match commodity hardware, spot instances, or specialized accelerators to suit your needs.
Future-Proofing with Open Standards and Frameworks
The AI/ML landscape evolves daily. Ensuring your stack can keep pace requires:
- Interoperable Model Formats
Standards like ONNX (Open Neural Network Exchange) liberate your models from single-framework captivity, ensuring you can deploy anywhere. - Open Container Standards
The Open Container Initiative (OCI) ensures your Docker images or equivalents run on any compliant runtime. - Kubernetes & Cloud-Native APIs
Kubernetes is open-source and widely embraced, giving you a stable baseline for orchestrating ML workloads across on-prem and multi-cloud. - Open ML Frameworks & Tools
PyTorch, TensorFlow, Hugging Face Transformers, Ray, these best-of-breed open libraries offer robust communities and vendor-agnostic capabilities. - Community and Consortium Efforts
Initiatives like the Linux Foundation's Open Platform for Enterprise AI (OPEA) promise collaborative, neutral governance, ensuring the AI stack remains open and composable.
Bottom line: Aligning with open standards is like buying “optionality insurance.” It guarantees your AI architecture will stand firm (and flexible) as the generative wave crests and reinvents itself, yet again.
Emerging Tools Enabling Open & Composable AI Stacks
A fresh wave of open-source projects is making it ever easier to adopt open, containerized pipelines:
-
vLLM (High-Performance LLM Serving)
An open-source inference engine that uses novel memory management (PagedAttention) to deliver huge throughput gains. It supports standard API endpoints and works across diverse hardware, letting you swap in your chosen LLMs without paying per-call fees to closed APIs. -
AIBrix (Cloud-Native Control Plane for LLM Orchestration)
An open-source toolkit layering atop Kubernetes to coordinate large-scale LLM serving (e.g., scheduling models, caching, autoscaling). It combines inference engines like vLLM with infrastructure logic so you can run fleets of LLMs in a cost-effective, fully containerized manner. -
KubeRay (Distributed ML on Kubernetes)
Ray is beloved for parallelizing Python-based ML tasks; KubeRay extends that power to Kubernetes. You can define distributed Ray clusters via custom Kubernetes resources, unifying your ML workflows (training, batch, inference) in one cohesive, autoscaling environment.
Together, these tools exemplify a “Lego set” approach: each piece is modular, composable, and swap-in-ready, ensuring you don't have to lock your entire pipeline around any single proprietary service.
Open GenAI in Practice: Real-World Success Stories
- Cost Savings & Performance Boosts
A gaming company combined open LLaMA models, model compression, and vLLM serving to slash GPU costs by half, while sustaining top-tier inference speed. - Multi-Cloud AI Deployment
Vodafone exemplifies a pragmatic anti-lock-in strategy by splitting data and model services across multiple clouds. This architecture gives them “flexibility and commercial control,” including the ability to route data from one cloud into a model running on another. - Enterprises Embracing Open-Source LLMs
Automotive marketplace Edmunds and airline EasyJet both reported success with open-source LLMs, citing benefits in security, flexibility, fine-tuning, and cost. - Multi-Model Strategies
Surveys show most enterprises now deploy three or more foundation models, mixing proprietary and open-source. This approach not only delivers better results (pick the right model for each job) but also evades lock-in risk by refusing to put all AI eggs in one basket.
Conclusion: Strategic Recommendations for CTOs
-
Prioritize Portability
Containerize everything; standardize model formats (e.g., ONNX). Ensure you can run your AI pipeline on any cloud, or on-prem, without rewriting code. -
Adopt Kubernetes as a Backbone
Establish Kubernetes (or an equivalent) for your AI orchestration. It's a proven, future-proof foundation. -
Leverage & Contribute to Open-Source ML
Use frameworks like PyTorch, TensorFlow, and Ray. Where possible, contribute enhancements, shaping tools to fit your business needs. -
Keep Your Stack Modular
Avoid all-in-one platforms. Break down your AI pipeline into microservices that can be swapped or upgraded independently. -
Plan for Multi-Model, Multi-Cloud
Don't rely on one LLM or provider. Design for easy model-switching, using open APIs and container orchestration to spread risk. -
Champion Openness Internally
Communicate the long-term strategic benefits of an open approach to the rest of the C-suite. Pilot projects that yield cost savings and agility wins will foster trust and adoption.
By combining open standards, containerized deployments, and modular designs, you and your enterprise can future-proof your AI efforts and remain masters of your own technological destiny. In a field evolving as rapidly as generative AI, that freedom is no small thing.
References & Further Reading
- AI Vendor Lock-In: Building Your House On Sand (LeanIX)
- Vodafone CTO Warns of Big Tech Lock-In with Generative AI (Light Reading)
- How Enterprises Are Using Open Source LLMs: 16 Examples (VentureBeat)
- Kubernetes & AI/ML Boosting Containerized ML Workloads 2024 (Easecloud.io)
- Unleash the Full Potential of LLMs: Optimize for Performance with vLLM (Red Hat)
- Introducing AIBrix: Cost-Effective & Scalable Control Plane for vLLM (AIBrix)
- Use Ray on Kubernetes with KubeRay (Google Cloud)
- What Every ML/AI Developer Should Know About ONNX (DigitalOcean)
- 2024: The State of Generative AI in the Enterprise (Menlo Ventures)
- Open Platform For Enterprise AI (Linux Foundation)